Agency MBS Bonds - Forward Looking No-Model Data-Driven Analytics
April 01, 2025
One argument for using models rather than historical data for bond valuation is that history does not repeat itself, and relying on it is like looking in the rearview mirror. Instead, we should polish our crystal ball and improve our forecasting models making our subjective assumptions even more complicated. But what do we do when models produce contradictory results - results that change over time and are not consistent, not even between different models, and sometimes not even between different versions of the same model?
In many situations, neither historical data nor excellent forecasting skills provide much help. A good example is economists' forecasts of economic indicators. As research shows, these forecasts are no better than random guesses around a consensus – which itself is not a good predictor of the released economic indicators.
However, in some cases, history can be a good predictor of the future – if you can identify historical relationships that are likely to persist or change slowly in the future, ideally lasting at least as long as your investment horizon. Some classical examples from the MBS (Mortgage-Backed Securities) market include:
- The relationship of prepayments vs. incentives and other collateral characteristics (S-curves)
- Credit transitions (the monthly probability of non-delinquent borrowers becoming delinquent)
- The impact of regulatory events (e.g., changes in LLPAs, MIPs, PLFs)
- The relationship between prepayments and HPA (Home Price Appreciation)
These relationships typically evolve slowly over shorter time frames but can shift materially over longer horizons (see Fig. 1) Therefore, as long as they remain stable over the investment horizon, they can be used for the forward-looking valuation but revisited longer term.
We believe that using this type of No-Model, Data-Driven Analytics is actually more forward-looking and accurate than relying on models built on subjective modelling forecasts (which vary by model and modeler which is typically less accurate). A few specific MBS valuation examples are below:
- Prepayments of collateral with similar characteristics in a similar environment (e.g., incentive; see Fig 3 – Base). The prepayment vector for G2 MB0208 (G2 7 Multi Feb Issuance pool) is obtained by breaking down its collateral into similar cohorts (e.g., by VA, FHA, loan size, loan purpose) at the current incentive level, and then using their prepayment vectors for valuation. This approach is statistically valid, as the relationship between prepayments and collateral characteristics is expected to remain stable over a typical investment horizon.
- HPA and Current LTV are major factors influencing mortgage prepayments (see Fig. 2). HPA has recently slowed compared to the past few years. So, what's the best way to value newly originated mortgage bonds? If we use prepayment speeds observed during a stronger HPA environment, we're once again looking in the rearview mirror. Instead, we only include in our data sample collateral with effectively lower HPA and higher Current LTVs. The impact on valuation of using lower HPA is material (see Fig 3 – 5% HPA).
- It is possible that the current administration could impose a Mortgage Insurance Premium (MIP) increase on FHA loans. Even though this hasn't happened recently, historical data can still provide insights into how such a change would affect prepayments if this happens. To see the valuation of the impact of this we just need to include FHA loans with lower incentives in our sample (see Fig. 3 – MIP + 30bps).
We strongly believe that valuation and risk analytics of MBS assets using No-Model, Data-Driven technology – avoiding subjective modeling assumptions and relying purely on data – is superior to subjective models. Moreover, this approach is consistent across all MBS asset classes, from securities to MSRs to whole loans.
There is a technical reason why models do not perform well for MBS: the MBS market is too diverse (or has too many degrees of freedom) for any model to describe it accurately. Making a model even more complicated only makes things worse, as it becomes harder to track without significantly improving accuracy.
Why Choose Us?
With over 20 years of experience and a proven track record in data and analytics development, IVolatility serves more than 500 institutional clients and 130,000 retail customers. Now, we're bringing this expertise to MBS data and analytics.
Discover the benefits of using IVolatility solutions for Mortgages:
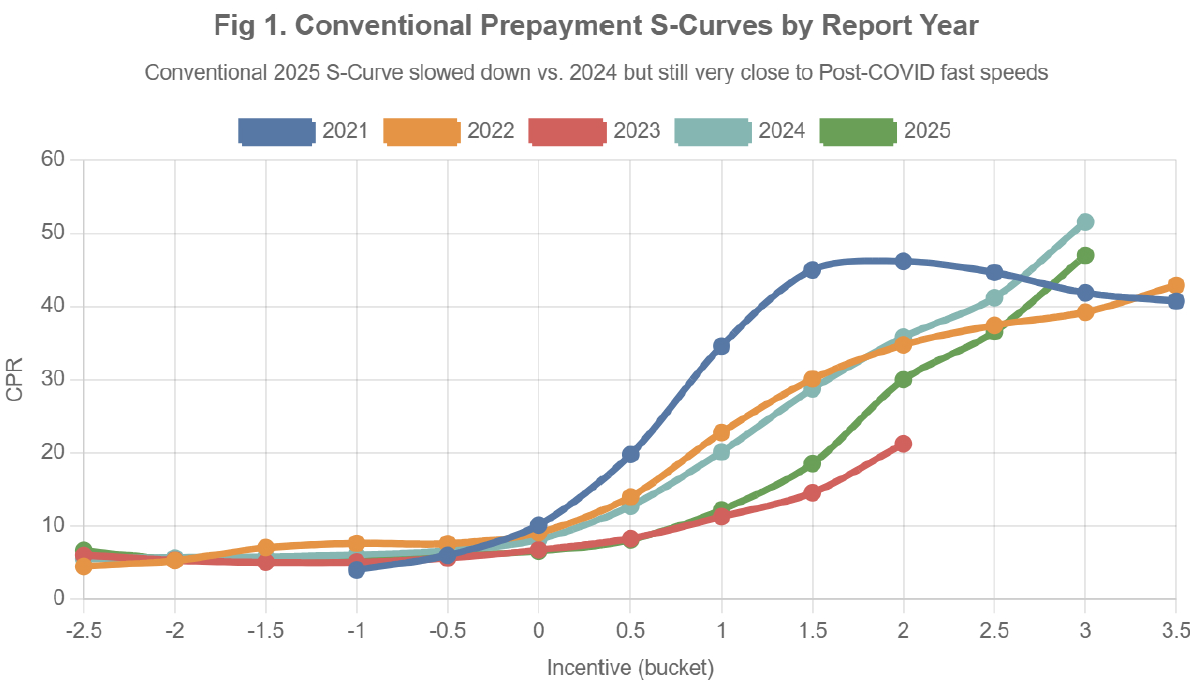
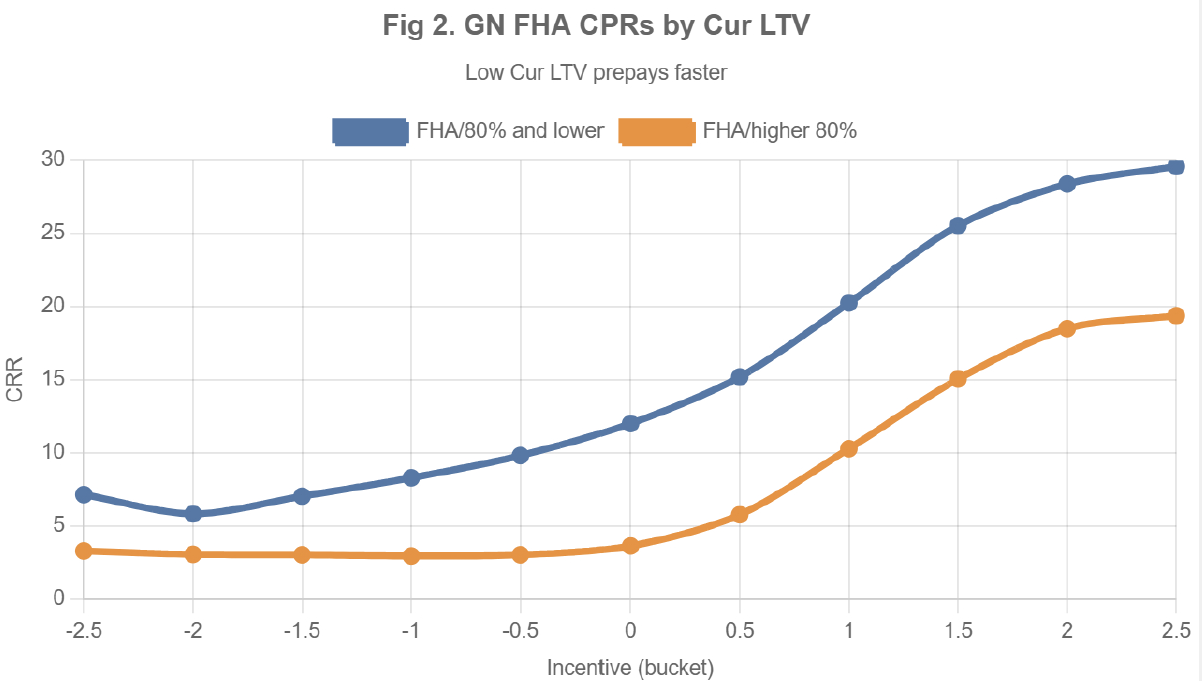
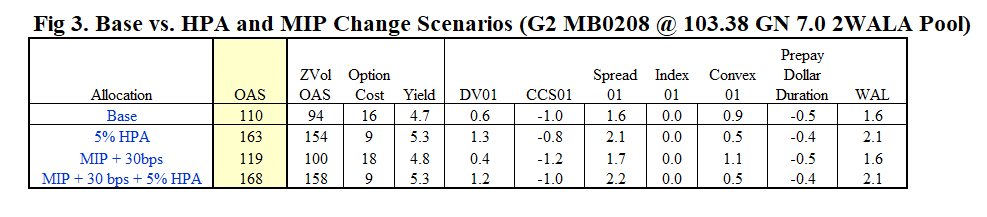
Got a question or a comment?
We're here to serve IVolatility users and we welcome your questions or feedback about the MBS data insights discussed in this post. If there is something you would like us to address, we're always open to your suggestions. Please let us know!
Previous issues are located under the News tab on our website.